8 Essential Elements for a Foolproof Data Governance Strategy

Data helps enterprises craft new business models, test growth strategies, and improve operational efficiencies. However, the staggering rate of data generation and scattered storage is a major bottleneck, making accessing data for business intelligence and regulatory compliance a recipe for disaster.
If your organization is facing similar challenges, it’s time to reassess your data governance efforts. With over 90% of companies failing in their initial data governance projects, you can learn from the fact that it takes an iterative approach to build an efficient data governance program.
This blog post covers the essential steps to help you steer your organization’s data governance efforts in the right direction. You'll also find at the end a FREE checklist to download for your internal use...
What is a Data Governance Strategy?
Data governance is a set of internal standards and policies designed to ensure data is used effectively and efficiently. The first step in understanding the typical data lifecycle (the stages that operate on the data) is to understand data governance strategies.
Data Lifecycle Stages
At a high level, the data lifecycle looks something like this:
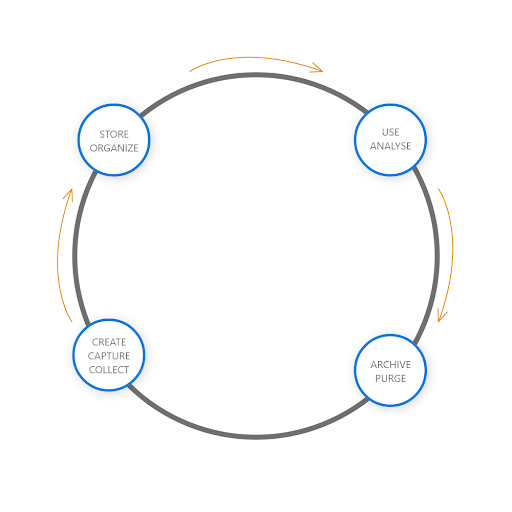
These stages are quite self-explanatory – it all starts with the “Create / Capture / Collect” stage and goes through “Store / Organize” and a series of “Use / Analyze” stages. Finally, the data ends up being archived or destroyed.
The stages may seem pretty simple at first sight. However, in an enterprise context, this is not exactly the case. That’s because the data from multiple sources are ingested and shared across numerous data pipelines. To facilitate these operations, the data lifecycle must have additional stages:
With this, you’ll likely end up with a fan-out scenario, in which multiple data pipelines share data across lifecycles.
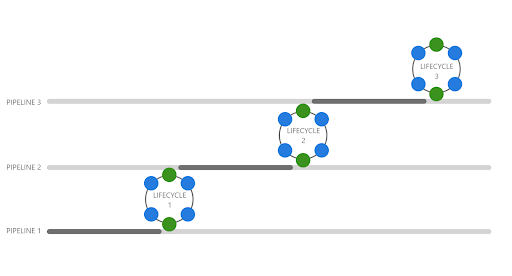
Things get complicated when this happens in parallel. When more people and tools are involved in managing data, there is every likelihood that you’ll lose visibility on data usage.
Therefore, data governance is essential for managing the growing complexity of the data lifecycle within a set of enterprise data pipelines. At its core, data governance unifies the People, Processes, and Platforms (the 3 Ps) to devise a set of policies for regulating data access. The end goal is to establish a set of rules to establish the 4 Cs, Coherence, Consistency, Compatibility, and Compliance, for every data access.
The 3-Ps are:
- People are the most frequent data manipulators. By enacting policies for data access by different people, you can control data sharing and manipulations.
- Processes define a series of actions taken on data, spanning across the data pipelines. Putting these actions under the boundaries of data governance ensures that only legitimate actions can be taken on the data.
- Platforms comprise software tools and infrastructure to store and process the data. Since many of these platforms operate on data in an automated way, data governance ensures that data access and manipulation by these platforms are benign and in line with the overall objectives.
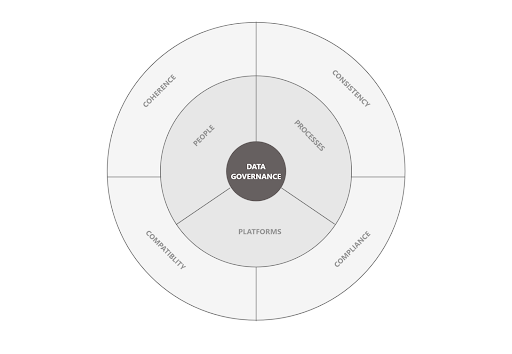
What are the Benefits of Data Governance?
- Coherence, meaning all points representing a dataset are captured, shared, and stored in the same structure.
- Consistency, to guarantee uniform format while accessing the same data across multiple mediums or data consumption interfaces.
- Compatibility, to ensure that all the data processing tools and platforms can access the data in the same formats.
- Compliance, to enforce strict data exchange boundaries that help you meet all the necessary government regulations.
Data Governance vs. Data Management
8 Essential Elements for a Foolproof Data Governance Strategy
Data governance applies to all business functions, so the rules of accessing and engaging with data across departments, business units, or functions can get complex and murky. If you are serious about overhauling your data governance practice, you’ll need to unpack and define these complexities.
Therefore, one of the core activities as part of a data governance strategy is to write and maintain a master strategy document. This document spells out the core elements in a way that standardizes access to data in order to satisfy the 3-Ps and the 4-Cs of data governance. Here are our six recommended elements that must be part of this document, plus two optional architectural approaches.
1. Data Cataloging
Data cataloging is the process of tagging data with additional information, making it easy to organize and manage data assets. It is done as part of the discovery phases of data governance, where you identify all the data created or ingested within the entire organization.
Once the data asset is identified, you can capture additional metadata for each dataset, such as categories, labels, or mappings to existing organizational jargon. These are then stored separately to build a data dictionary, with a reference to the actual data source, for easy identification and retrieval.

2. Data Lineage
The function of a data governance program is incomplete without the ability to tackle anomalies, which range from unauthorized access, policy violations, or data quality issues leading to a potential business impact. Data lineage establishes the history and tracing mechanism for every data lifecycle across the 3-Ps. It plays an important part in gathering evidence to help the data governance teams identify the root cause and take corrective actions.
3. Data Standardization
Data standardization ensures that data formats are consistent across the organization. It applies at the datum level, as well as larger data. For example, at the datum level, you could format an employee name field based on the sequences of first, middle, and last names, which must be standardized. Similarly, at a macro level, data formats must be standardized so creators and consumers of the data can collaborate seamlessly.
An exhaustive set of data standards also addresses some of the common data normalization problems, such as blank data fields or invalid formats. Together with data cataloging, data standardization addresses the quality and access to data and forms the basic requirement to achieve data coherence.
4. Data Access Management Roles
Access management defines the policies for users, groups, and roles that have access privileges to data. You can further granularize access privileges based on actions that are allowed or barred on the data, such as creation, modification, and deletion.
One of the main challenges of defining data access management policies is the wide range of technologies and platforms where data is scattered. For example, some data is located in the databases, whereas some are ingested from event sources, APIs, or third-party applications. That's why you need to define key roles to safeguard access and ensure the quality of data.
5. Data Archive / Purge Policy
Data archive and purging essentially means cleaning up your business’ old data. These processes bring the data lifecycle to a final conclusion – when you no longer need the data, what should you do with it? You can consider a couple of special situations for data archiving and purging:
- Dark data: Organizations generate a lot of dark data that is never used but contains critical or sensitive data. The policies must mandate how to handle these forms of data.
- Temporary data: Often, a lot of temporary data is generated from ad-hoc activities that must be properly disposed of.
6. Data Compliance
Compliance is an often ignored aspect of data, so it’s a good idea to have a separate section in your data governance strategy document to define key policies for data compliance. It’s an essential step for meeting regulatory requirements and attaining data and information security certifications, such as GDPR and ISO 27001. It’s worth noting that you can leverage data cataloging to add additional labels for compliance purposes to help identify data that is a potential target for compliance issues.
7. Data Mesh
A data mesh is an evolution of the traditional big data architecture that splits the centralized data architecture into a decentralized arrangement, in which separate data teams are established based on domains. These domains are categorized into corporate functions, such as sales, marketing, or customer service.
From a data governance perspective, data mesh creates independent data ownership with a single source of truth and a single point of access. Therefore, it’s worth splitting all the above elements of your data governance strategy document into multiple data domains, based on the challenges your organization faces in data administration.
8. Data Fabric
.png)
A data fabric integrates all the data and analytical processes to allow frictionless access to data across multiple distributed data stores. It acts as a central data management platform overseeing the correct usage of data assets across your entire organization.
Data fabric is optional and might not be part of your initial data governance strategy. However, as the data volume increases, data fabric is a valuable addition to streamlining the data management tasks under the direction of your central data governance team.
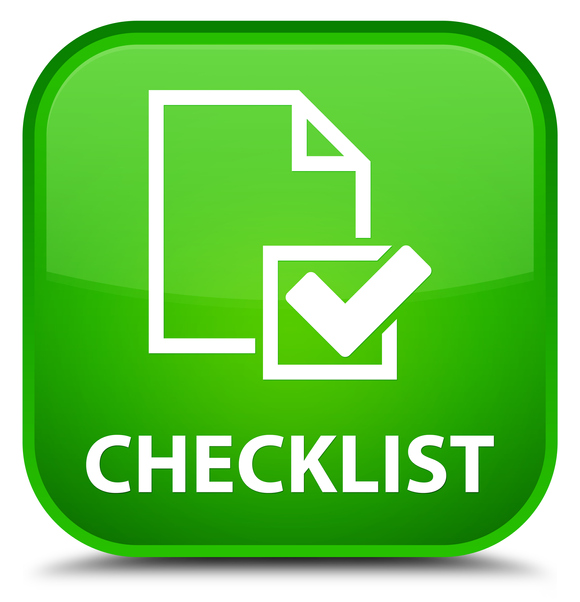
Maximizing the Value of Data Governance Through Data Management Solutions
The ultimate aim of data governance is to foster better interaction among data owners, data creators, and data consumers. Each element in the data governance strategy document is meant to streamline this interaction, one step at a time. Aggua’s data governance augmented data management platform offers both features in the same hub, enabling business teams to access data and practice data-centric decision-making while maintaining the sanctity of the data governance strategies. Manage the availability, visibility, usability, security, and integrity of your data in one single source of truth.