7 Ways to Leverage AI for Data Analytics

The marriage between artificial intelligence and data analytics is a match made in data engineering heaven. Without machine learning and AI, data analysts and engineers would waste time looking for needles in spreadsheet haystacks. With rapid developments in AI technologies and capabilities drawing more public attention, there is an AI solution for (almost) every use case and industry, from education to logistics.
How does the global adoption of AI in business applications impact the field of data analytics? And how can you leverage AI in data analytics to create positive business outcomes in 2023 and beyond? Let's find out.
What is Data Analytics in Business?
Data analytics is the intricate and diverse science of mining large data sets to discover patterns, deliver quick answers to structured queries, and draw conclusions. In business, data analytics means extracting actionable insights from interconnected data sets, leading to better decision-making in fast-paced and competitive markets.
Data analytics aims to enable organizations to explore all their data (real-time, historical, unstructured, structured, qualitative) to identify patterns and generate insights to connect business intelligence with action. You can categorize data analytics processes into four main types:
- Descriptive analytics - Studying past events over a period of time to discover trends and patterns. For example: Have the sales increased compared to last month?
- Diagnostic analytics - Finding out why something happened by adding more data. For example: Did the growth in website visits increase conversions? Did the latest marketing campaign impact sales?
- Predictive analytics - Attempting to foresee what will likely happen in the near term based on past data and additional input. For example: How did previous marketing campaigns impact website views and sales? Which channels used in the past are most likely to provide an increase in ROI?
- Prescriptive analytics - Suggesting a course of action based on available data. For example: If the most effective channel for high lifetime value user acquisition in the past three campaigns was LinkedIn, how much of the budget should we allocate to it and other channels in the next campaign?
What is Artificial Intelligence in Data Analytics?
Manual data analysis is not only time-consuming and labor-intensive, but also inefficient and lacking in accuracy. Unlike humans, machines excel at analyzing and monitoring large volumes of distributed and unstructured data. It turns out that machines in 2023 are pretty good at it, too.
AI in data analytics empowers data engineers to automate and enhance various aspects of data analysis. These include data preparation, insight generation, pattern recognition, and more.
Natural language processing (NLP) and machine learning (ML) models are two of the main AI technologies often employed in business data analytics. While ML identifies patterns in historical data and uncovers relationships between data points, NLP can convert the conclusions into human-readable insights.
What are the Benefits of AI for Data Analytics?
Long before ChatGPT was the leading buzzword of the tech industry, AI was transforming the field of analytics. Bots and scripts today offer speed, scale, and granularity that isn’t humanly possible. And all at a fraction of the cost of a data team, including humans, that can crunch numbers with applied mathematics and statistical analysis models.
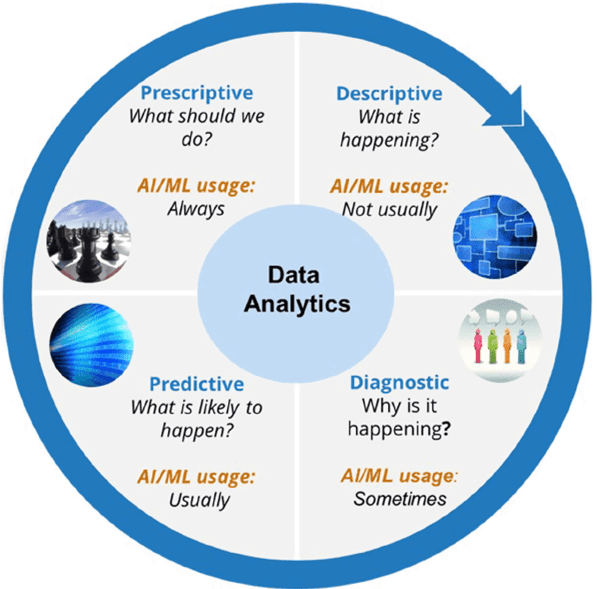
AI enhances data analytics by drastically improving it through automation. It makes data analytics more accessible to various stakeholders through improved user interfaces and more powerful by enabling the contextual and near-real-time analysis of versatile and unstructured datasets.
How Can AI Help Prepare Your Data for Analytics?
Your data analytics must rely on accurate and prepared data for truthful and insightful outcomes. The process of data preparation entails the discovery, cleansing, transformation, and enrichment of your data. For example, the data preparation process can include standardizing data and currency formats, enriching source data, removing outliers, and ignoring duplicate records.
Data preparation is vital to any data analytics project or task for contextualizing, verifying, and organizing data before it can be turned into accurate insights. Thorough data preparation enables efficient data analysis, reduces errors and inaccuracies, and turns the processed data into a resource accessible to relevant data consumers.
Data preparation is often regarded as a lengthy and tedious undertaking for data engineers or business users. However, with advanced data fabric-augmented AI platforms like Aggua offering data quality and observability solutions, you can automate the detection and correction of anomalies in your data and test your data and meta-data from the source, through your ETLs up until your BI and Dashboards.
7 Business Use Cases Where You Can Leverage AI for Data Analytics
The potential applications of AI in data engineering and data analytics specifically are almost boundless. Turning big data into value with AI technologies highly depends on where artificial intelligence can do things humans cannot, or what repetitive tasks it can offload. Below are just a handful of examples of how businesses can leverage AI-powered data analytics to drive business growth and reduce business risk.
1. Customer behavior analytics & UX hyper-personalization
AI data analysis tools can help acquire, retain, and grow client value by collecting user information and engagement data (CDP) from multiple sources (e.g. CRMs, website and app analytics tools, third-party data, etc.) and then using it to augment and enhance the buyer journey and customer experience overall.
This unified analysis of data sources also enables businesses to create focused and hyper-targeted marketing and advertising campaigns, leveraging AI-generated insights to gain higher ROIs.
2. Business intelligence & pricing analytics
Understanding and acting upon the behaviors of your customers and prospects is one side of the coin, while the other is near-real-time competitive intelligence and trend forecasting. For example, Amazon sellers can leverage AI to increase their revenues and product performance through actionable content and pricing suggestions.
3. Inventory & production optimization
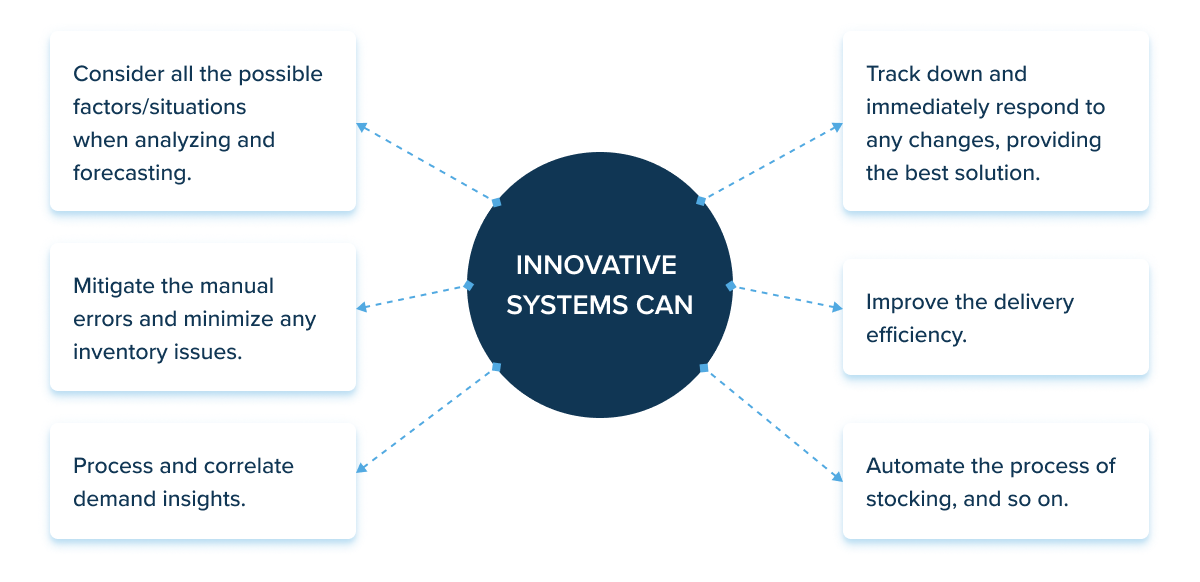
Inventory stocking depends highly on data from marketing, sales, purchasing, and production departments. You can apply AI algorithms to process all that data and implement optimization of production volumes and inventory levels. This way, retailers can confidently invest in ad campaigns for the product with the highest stock levels and shortest acquisition or manufacturing periods.
4. Operational efficiency in logistics
AI analytics can be applied to most business operations including optimizing supply chains and applying data-driven knowledge to find optimal solutions to previously unsolvable problems. It can help optimize rotas and schedules, monitor critical processes, and perform predictive maintenance in manufacturing.
5. Fraud detection
AI can gather user behavior analytics by monitoring websites, applications, and services to detect suspicious activity. In addition to detecting fraud, AI data analytics can aggregate data from multiple sources to build profiles of “normal” behavior at peacetime to reduce false positives and increase accuracy.
For example, digital businesses can pre-empt phishing and brand impersonation attacks on their websites by employing AI-based real-time monitoring that works behind the scenes to detect attempts to clone or exploit their authentic digital assets.
6. Cybersecurity
With the help of AI analytics and generative AI tools like ChatGPT, security alerts can be contextualized, verified, and sorted by severity, even when dealing with complex multi-cloud and containerized infrastructure.
7. Root cause analysis
AI-driven analytics effortlessly digs through vast volumes of data to map out correlations between incidents and their primary causes. This includes complex data pipelines and ETLs, where AI-enhanced data analytics can help pinpoint and resolve issues with little to no manual work.
Improve Your Data Analytics and Enable Efficient Data Management with Aggua’s AI Capabilities
Data analytics is one of the fields that has the most to gain from the technological advancements and increased popularity of artificial intelligence. The applications of AI in data analytics are almost endless. Aggua is constantly developing new technologies to stay up to speed with market trends and innovations like AI and ChatGPT. Look out for some exciting product announcements very soon!
Aggua is a data fabric-augmented AI platform that gives data and business teams access to practical data insights for more holistic and data-centric decision-making. Our best-in-class lineage mapping gives you a holistic view of your entire data pipeline, and you can easily search your entire data universe and see cost and performance findings (and more) in Aggua’s one-stop-shop Data Catalog.
To dig deeper into your costs, you can get granular information with our first-of-its-kind Cost Management & Optimization solution, which helps you identify exactly where and how to save on your data spend. Using AI capabilities, a recommendation engine will analyze your cost across the entire lineage and suggest methods in which you can optimize your spending based on usability.